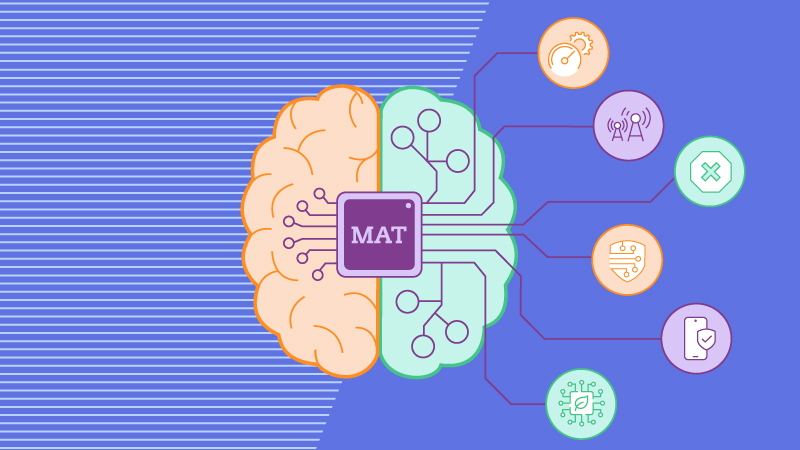
6 uses cases of machine learning
Automation and machine learning are transforming the world of telecommunications, and 2023 is the year of machine learning, as explained in the post “The Time Of Machine Learning Is Now”. This allows companies in the sector to make faster, more efficient, and more accurate decisions and provide a better customer experience. Below are six use cases we have seen work successfully in different CSPs worldwide.
Anomaly Detection: With machine learning, automating and improving network fault detection is possible. Anomaly detection algorithms are used to identify data points that significantly deviate from expected patterns in the data. These algorithms can be used to detect faults in the network by analyzing various performance metrics, such as network traffic, resource utilization, and error rates. In this scenario, the machine learning algorithm is trained on a large data set of the network’s normal behavior. The algorithm uses this training data to create a model representing normal behavior. This model is then used to identify any anomalies or deviations from normal behavior in the network data in real time. For example, suppose the network experiences an increase in error rates or a decrease in network performance. In that case, the algorithm can detect this as an anomaly and generate an alert. This allows network administrators to quickly identify and resolve the problem before it becomes a major issue, reducing downtime and increasing network reliability.
Optimization of Network Planning and Deployment: Machine learning can help telecommunications companies analyze large amounts of data to identify the best locations to deploy 4G/5G cells in a Radio Access Network or FTTH OLT for a Fixed Access Network. Using information from GIS-provided vector values, data from existing customers in our CRM, potential customers provided by market intelligence reports, weather history, and availability of physical space to deploy equipment for each region, combined with automation that initiates the purchase, installation, configuration, commissioning, integration, and acceptance process, we can have a more agile network deployment, which translates into greater efficiency and reduced time to market.
Detection and Prevention of Telephone Fraud: Machine learning can detect patterns of fraudulent calls using CDR and CRM as sources of information. With machine learning, we can avoid false positives resulting from static and general rules. Patterns can be specific to each user, considering their behavior history and users with similar characteristics, allowing for more granular detection and, therefore, more efficient detection. Combined with automation that proactively closes that traffic and alerts the NOC and customers, we can generate an end-to-end use case that generates significant savings and value for our customers.
Network Capacity and Growth Analysis: With the exponential growth of traffic in networks, the waiting times for traffic forecasting and growth planning have to be reduced to find a sweet spot where the time from ordering new hardware to installation and commissioning is imperceptible to end-users. Engineers have to manually analyze traffic curves at regular intervals, trying to identify trends and anticipate saturation. Many cases use static thresholds depending on the company’s standards (70%, 80%, 90%), which can also vary depending on the region and the type of service. Using a trained model, we can automatically identify traffic patterns, taking into account the equipment and the interface’s particular characteristics.
Energy savings: Mobile access networks are a critical component in the telecommunications infrastructure and require high energy consumption to function effectively. In order to reduce costs and be more sustainable, CSPs are looking for ways to optimize their networks’ energy consumption. Machine learning can solve this problem by allowing the analysis of large amounts of energy consumption and network traffic data to identify patterns and trends. The algorithm can analyze the behavior of an entire city, including all sites, cells, frequencies, and users, thus allowing to detection of optimization opportunities that do not affect the quality of service perceived by customers. Combining it with automation, we can generate an end-to-end use case where sector shutdown is done unattended, allowing these savings to be executed 7x24x365. This allows CSPs to make informed decisions about network configuration and usage to reduce energy consumption, resulting in significant savings in operating costs and a reduction in their carbon footprint.
Detection and Prevention of Security Incidents: Security is a major concern in telecommunications, as networks and user data are vulnerable to security threats such as DDoS attacks or phishing, to name a few. Detecting and preventing security incidents is essential to ensure the network’s protection and user data’s privacy, and machine learning is a valuable tool in this critical task. Machine learning can help CSPs detect and prevent security incidents before they occur or at very early stages where the impact is still low. By using machine learning algorithms, companies can analyze large amounts of security data in real-time to identify patterns and trends that indicate a possible attack. Once a threat is detected, automated systems can take measures to prevent it and notify the network security team.
These six use cases generate savings and efficiency, improve the customer experience, and provide new opportunities for CSPs. As a senior telecommunications engineer, these machine learning use cases can significantly impact the telecommunications industry. I look forward to seeing them in action. It is essential to generate momentum, and I hope that some of them inspire you to implement them in 2023.