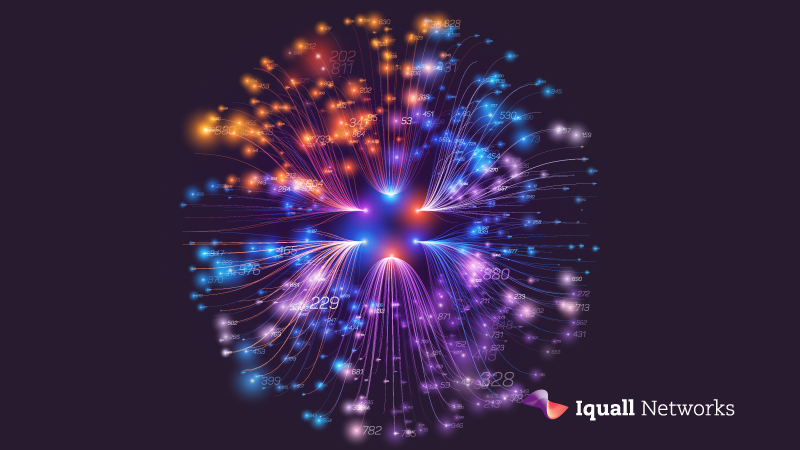
Demystifying Machine Learning in Telecommunications
Anyone who has spent long hours and days analyzing statistics to understand why a particular quality, traffic, or general behavior indicator in the mobile network has changed outside its normal behavior will appreciate the advent of Machine Learning techniques. These are not only useful for detecting these deviations but also for correlating this anomalous behavior with its possible causes.
However, it’s essential to demystify the belief that Machine Learning is miraculous, learns on its own, and will magically provide solutions. Focusing on network analysis, I limit Machine Learning to supervised learning techniques. Supervised learning involves analyzing the historical behavior of a specific indicator or set of indicators (input variables or features) and applying a function (model) to predict how the indicator under analysis (output variable or labels) will behave.As mentioned, the function or model to which I refer is not always trivial, and this is where various techniques and algorithms come into play to help decipher it.
Another aspect where Machine Learning and the automation of the aforementioned process helps is that it allows me to multiply my analytical power as many times as defined, add as many input variables as considered necessary, and include in the Machine Learning process (applying the right techniques) the reduction of input variables to retain the most representative ones that explain the behavior of the output variable to a high degree.
For the Machine Learning process to scale, endure, and truly become part of the day-to-day operations of the requesting area, it should not be the initiative of a single collaborator who understands Machine Learning techniques, takes a dataset, processes it, obtains a result, and lists variables that are outside the expected behavior. The key is to automate the process, starting with the systematic collection of data, refining it, parameterizing the algorithms of Machine Learning techniques, running the model for the predetermined data set, validating the model, preserving each validated model in a new version of CI/CD, and incorporating as part of the process the continuous evaluation of the model, initiating new parameterizations of the model when the current one exceeds the maximum expected error, or triggering an alarm when the automatic adjustment of the model does not meet the predefined error threshold as acceptable.
This process is what we call Machine ML Ops, incorporating Machine Learning within the DevOps process. Once confidence in automatic detections is achieved, it becomes very simple to take the next step and incorporate the results of Machine ML Ops into the network’s remediation or adjustment loop to maintain the indicators at values that satisfy the required range to ensure quality.
In conclusion, the integration of Machine Learning into network operations is not just about leveraging advanced technology; it’s about fundamentally transforming how we approach problem-solving in the telecommunications sector. By embedding Machine ML Ops within our processes, we not only enhance our analytical capabilities but also pave the way for more proactive, efficient, and accurate network management. This evolution marks a significant step towards a future where network reliability and optimization are not merely goals, but assured realities, driven by the intelligent application of Machine Learning techniques.
By Elias Barmak, Presales specialist of Network Automation at Iquall Networks
https://www.linkedin.com/in/ebarmak/