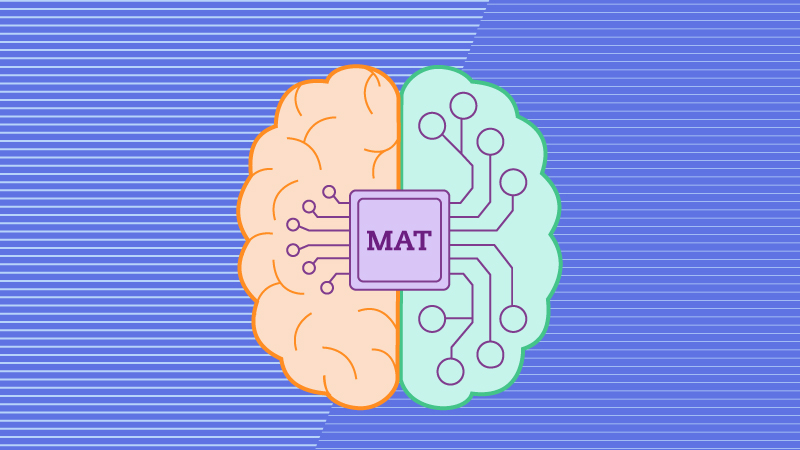
The time for machine learning is now!
¿Where we are?
We are starting 2023 and yet, there are many CSPs that have a low level of adoption of Machine Learning, but mainly very heterogeneous. In the same company, we can find the latest advances in artificial intelligence and processes that are executed in the same ways as they were executed 10 years ago. It becomes harder and harder to keep a service assurance process, compliance of health, sustainable in time if we do it in a traditional way. As new technologies generate new opportunities, not incorporating these new technologies such as Machine Learning may lead to losing those opportunities to traditional or emerging competitors.
It is nothing new to say that the big challenge is data governance. There are endless sources of truth with different standards, and in many cases, without a definite scheme to articulate needs and capabilities needed in a dynamic business. Many of the projects that were the origins of those sources of truth were implemented in different times, with different objectives and standards, and we can find many of them in an intermediate stage in which they can add value but without reaching all their potential.
Even though CSPs have a considerable amount of resources, they sometimes need to use them to meet many simultaneous demands. All the CSPs we are continuously in contact with are looking for a way to implement it massively, but the real challenge is to set the wheels in motion. I will try to provide three arguments when it comes to making a decision on which projects to prioritize among many important and urgent ones.
1. The cost-benefit balance is at its best
Implementing Machine Learning today is relatively fast and cheap. I say “relatively” because it depends on the moment our company is regarding the reskilling/upskilling and technical debts. Be that as it may, there are specific use cases that have the potential to generate value rapidly and with such a big benefit that allows us to repay them with very little effort. It is important to limit the scope and understand that the most important thing is to build up momentum to solve the equation in an agile manner and getting backup from our sponsors and technical teams without being frustrated in the process.
2. It allows us to grow exponentially
Incorporating cutting-edge technology to our processes allows us not only to rethink and improve them but also to continue growing exponentially without the need of a lineal change of our devices especialy in 5G, SDWAN & IOT networks. Technology and business are accelerating so that, in ten years time, they will have unprecedented speed and reach. Sustainability will depend on our capacity to run those businesses with highly competent, professional and agile teams that have the tools to generate value in a constant and exponential manner.
3. It builds the basis for the future
Machine Learning is a kind of artificial intelligence with specific applications. It has several success cases in practically all the industries and it is capable of generating value in the short term. But it is also a big first step towards new implementations of Artificial Intelligence. Overcoming governance and reskilling/upskilling challenges, rethinking our projects and schemes and most importantly, generating a new organizational mindset can let us move faster than our competitors in what is to come.
Only 3?
There are many additional reasons why we should raise our bets on machine learning and make it the core of the value generation strategy for this 2023. I chose the 3 that I consider most compelling to take to a strategy table where the key players are who determine the focus that aligns the work teams allowing them to achieve the annual objectives. We have not talked about some concrete use cases and how to generate a continuous cycle of value using Machine Learning, also known as MLOps, but that is a topic for the next blog entries